Caiwen Ding
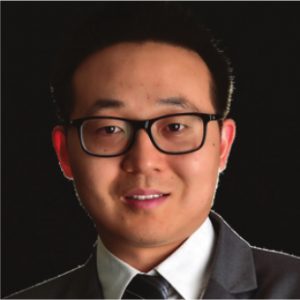
Congratulations Caiwen Ding on receiving a National Science Foundation CAREER Award for his proposal titled “CAREER: Algorithm-Hardware Co-design of Efficient Large Graph Machine Learning for Electronic Design Automation”. The goal is the project is to address the efficiency and scalability of using graph learning for Electronic Design Automation, thought a series of algorithm-hardware codesign approaches.
Caiwen Ding is an assistant professor in the School of Computing at the University of Connecticut. He received his Ph.D. degree from Northeastern University (NEU), Boston in 2019, supervised by Prof. Yanzhi Wang. His interests include Algorithm-system co-design of machine learning/artificial intelligence, privacy-preserving machine learning, machine learning for electronic design automation (EDA), and neuromorphic computing. He is a recipient of the 2024 CISCO Research Award and NSF CAREER Award. He received the best paper nomination at 2018 DATE and 2021 DATE, the best paper award at the DL-Hardware Co-Design for AI Acceleration (DCAA) workshop at 2023 AAAI, outstanding student paper award at 2023 HPEC, publicity paper at 2022 DAC, and the 2021 Excellence in Teaching Award from UConn Provost. His team won first place in accuracy and fourth place overall at the 2022 TinyML Design Contest at ICCAD. He was ranked among Stanford’s World’s Top 2% Scientists in 2023. His research has been mainly funded by NSF, DOE, DOT, USDA, SRC, and multiple industrial sponsors.
Abstract: Estimating Power, Performance, and Area (PPA) earlier in the electronic design automation (EDA) flow would improve the Quality of Results (QoR) and reliability in chip design. The classical analytical or heuristic methods can be challenging to fine-tune, especially for complex problems. Machine learning (ML) methods have proven to be effective in addressing these problems. Graph Neural Networks (GNNs) have gained popularity since they are among the most natural ways to represent the fundamental objects in the EDA flow. However, with increased design complexity and chip capacity, an increasing performance gap exists between the extremely large graphs in EDA and the insufficient support from general-purpose hardware, such as mainstream graphics processing units (GPUs). This project aims to expedite the large graph machine learning on various EDA tasks, through a full-fledged development of efficient and scalable computing paradigms. This project's novelties are EDA domain knowledge-aware graph machine learning, training acceleration, and algorithm-hardware co-design and optimization. The project's broader significance and importance include: (1) to advance the field of machine learning in chip design, highlighted in National Artificial Intelligence Initiative; (2) to deepen the understanding of interactions among EDA domain knowledge, graph learning, and GPU acceleration; (3) to enrich the computer engineering curriculum and promote participation from undergraduates, underrepresented groups, and K-12 students in STEM fields through relevant programs.
Dongjin Song
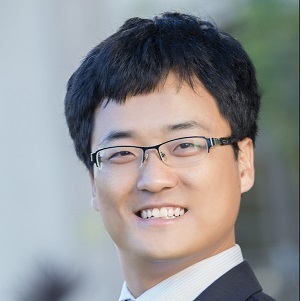
Project Framework
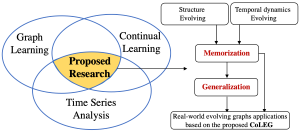
Congratulations Dongjin Song on receiving the prestigious National Science Foundation (NSF) CAREER Award to support his research project titled "CAREER: Towards Continual Learning on Evolving Graphs: from Memorization to Generalization". This project will develop a generic machine learning paradigm, Continual Learning on Evolving Graphs (CoLEG), to resolve the catastrophic forgetting problem by retaining essential structural information and temporal dynamics, ensure the generalization capability, and address real-world applications on evolving graphs. Specifically, he not only plans to tackle the catastrophic forgetting issue in structural evolving graphs via graph sparsification and topology-aware embedding, but also aims to develop new algorithms to incorporate structural and temporal dynamic patterns of evolving graphs under different regimes, resolve the task-free challenge, and reveal high-order dependencies. He will also develop novel solutions to pursue and imp pre-trained models and facilitate test-of-time adaptation to ensure the generalization over unforeseen scenarios.
Dongjin Song has been an assistant professor in the School of Computing, University of Connecticut since Fall 2020. He was previously a research staff member at NEC Labs America in Princeton, NJ. He received his Ph.D. degree in the ECE Department from the University of California San Diego (UCSD) in 2016. His research interests include machine learning, data science, deep learning, and related applications for time series data analysis and graph representation learning. Papers describing his research have been published at top-tier data science and artificial intelligence conferences, such as NeurIPS, ICML, KDD, ICDM, SDM, AAAI, IJCAI, ICLR, CVPR, ICCV, etc. He is an Associate Editor for Neurocomputing and has served as Senior PC for AAAI, IJCAI, and CIKM. He received the prestigious NSF CAREER award in 2024 and the UConn Research Excellence Research (REP) Award in 2021. He has co-organized the AI for Time Series (AI4TS) Workshop at IJCAI, AAAI, ICDM, SDM, and MiLeTS workshops at KDD.
Abstract: In the modern big data era, data often grows continuously and its interconnections and temporal dynamics evolve. To cope with the continuous evolution in data, an intelligent agent needs to incrementally acquire, perceive, accumulate, and exploit structural and temporal dynamic knowledge throughout its lifetime. This project aims to develop a generic machine learning paradigm to conduct Continual Learning on Evolving Graphs (CoLEG). The success of this project will 1) benefit critical infrastructure (such as social networks, transportation, and renewable energy) and human welfare (in the form of, for example, improvements in healthcare and epidemiology), 2) provide an ideal platform for composing the areas of graph representation learning, time series analysis, continual learning, and causal analysis, and 3) develop open-source tools for evolving graphs that can advance diverse topics such as node classification, link prediction, and temporal forecasting, improve our knowledge of the physical world, and contribute to real-world applications. This project will also 1) engage high school students in research and outreach to K-12 teachers and students, 2) broaden the participation of underrepresented groups especially female and low-income students in STEM, and 3) educate undergraduate and graduate students through the development of new course modules in data mining and machine learning.