The Connecticut Advanced Computing Center (CACC) will host the annual CyberSEED event online on March 8th
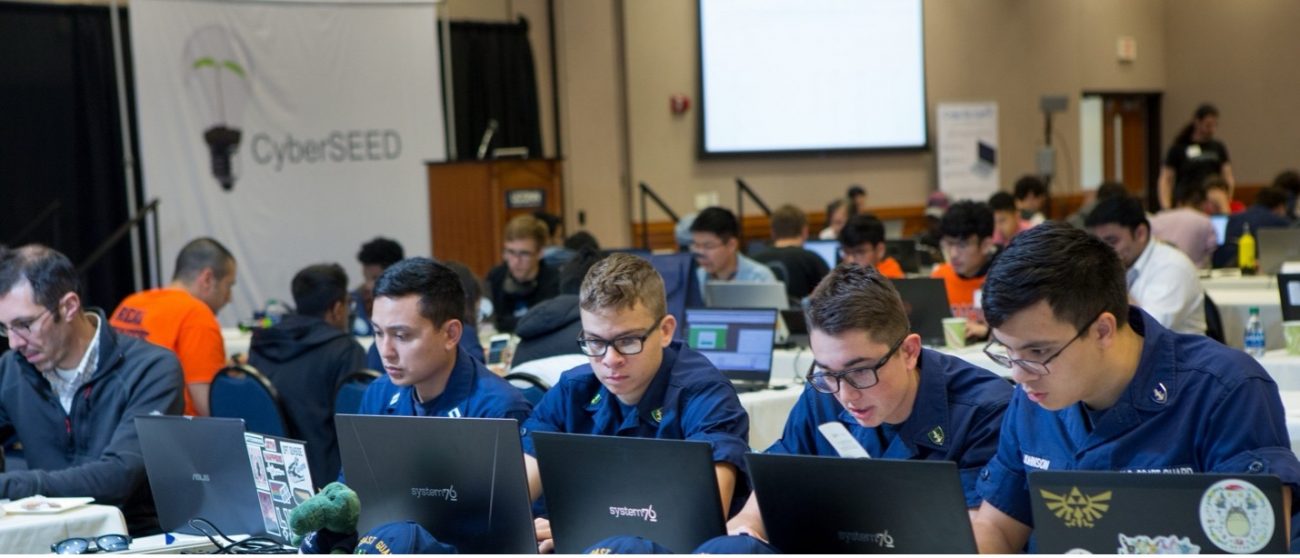
Welcoming teams from dozens of colleges and universities, CyberSEED’25 will feature a capture the flag event designed specifically for students. The Connecticut Advanced Computing Center (CACC) is hosting the annual CyberSEED event on March 8. The event will take place online.
Register for the event by 9 a.m. on March 8.
The competition will touch on a variety of cybersecurity challenges including a set of flags focusing on reverse engineering, web application security, network traffic analysis, cryptography, and more. The competition requires a breadth of skills, as well as scripting and programming alongside a solid understanding of network monitoring and forensics tools.
Each top-placing team is eligible to win prizes in amounts ranging from $3,000 to $250.
During last year’s CyberSEED event, the UConn Cyber team, ERROR 404, placed second out of 165 teams.
In response to their achievement, the club’s president, Anthony Crisci, said “We beat colleges with way better cybersecurity funding, courses, and just generally better way better cybersecurity programs than UConn. It feels good and hopefully helps bring more attention to, in my opinion, one of, if not the most important area of technology.”
Laurent Michel is a computer science and engineering professor and organizes the CyberSEED event.
“CyberSEED is a wonderful opportunity for aspiring CyberSecurity Professionals and I’m thankful for Synchrony’s support throughout the years,” Michel says. “UConn’s own teams participated in CyberSEED’24 and the ‘ERROR-404’ teams distinguished itself by securing second place on the roster. I look forward to the outcome with this new group of students and wish them all the best in walking in the footsteps of the other Huskies who did so well. Go Huskies!”
The competition platform is provided by Cyber Skyline and the event is generously sponsored by Synchrony.
Event Details:
This event will be hosted online. Organizers will be using Zoom to coordinate and communicate with all teams during the event. During registration, students should complete the CyberSEED Liability Waiver and email completed forms. Students must register as a team, and should come prepared with a laptop.
Orientation will take place at 9:30 a.m., actual kick off will begin at 10:00 a.m.