Congratulations Shaoyi Huang on your paper privacy-preserving machine learning acceleration being accepted for presentation at NeurIPS 2023.
Can you summarize your research area?
My research focuses on efficient machine learning on general AI systems, including efficient inference and training algorithms, algorithm and hardware co-design for AI acceleration, energy-efficient deep learning and artificial intelligence systems and privacy preserving machine learning.
What is the overarching goal of your graduate study?
My graduate studies are dedicated to spearheading the development of efficient machine learning, focusing particularly on addressing the computational and energy challenges of Deep Neural Networks (DNNs). My objective is to develop cutting-edge solutions in model compression and efficient training algorithms, alongside optimizing system design. The aim is not only to improve the performance of DNNs but also to reduce the environmental footprint of their training and inference process.
My approach is characterized by an intensive investigation into model compression and sparse training techniques, optimization algorithms, and the synergy between algorithms and diverse hardware platforms, which includes GPUs, FPGAs, and emerging technologies like ReRAM. The goal is to catalyze the emergence of neural networks that are not just sustainable and scalable, but also democratically accessible and ethically responsible.
How do you hope that you will have changed computing in five years?
In the next five years, I am committed to continuing my work in the field of efficient machine learning and AI systems. My objective is to spearhead a series of breakthroughs, particularly in enhancing energy efficiency—a cornerstone for sustainable technological advancement. Through an integrative approach of algorithm and hardware co-design, I foresee my efforts contributing to more synergistic and robust AI systems.
The democratization of AI is another pivotal aspect of my vision. I intend to break down barriers, making sophisticated AI tools accessible to a wider audience and facilitating their integration into a myriad of devices. By doing so, AI will not only serve the few but empower the many, transcending traditional technological limitations.
Moreover, my enthusiasm for refining the intricacies of large language models and generative AI is unwavering. These areas are ripe with potential to revolutionize how we interact with and benefit from artificial intelligence. By fostering innovative algorithm development alongside hardware co-design, I am confident that we can make AI use more sustainable, ethically grounded, and impactful.
My aspiration is not merely to advance the field in academic or technical terms but to ensure these improvements lead to tangible benefits for society. By driving these changes, I hope to play a part in shaping a future where AI is not only more efficient but also more aligned with the ethical and practical needs of our global community.
How does additional support allow you to more effectively complete your graduate study?
During my Ph.D. study, besides the mentorship from my advisors Prof. Caiwen Ding and Prof. Omer Khan, I received multiple forms of additional support, such as fellowship from the Computer Science Department, CACC, Cigna, Eversource and Student Travel grant from Workshop for Women in Hardware and Security, advanced computational resources from the lab. They are instrumental in enhancing the effectiveness and scope of my graduate research, pushing me to a higher level. Financial assistance alleviates the burden of tuition and living expenses, enabling me to dedicate more time to my studies and research, delving deeply into complex problems and innovating in the field of efficient machine learning. Access to state-of-the-art GPUs allows me to experiment with large-scale models and datasets, conduct extensive experiments and simulations and verify the effectiveness of designs more rapidly. This is particularly crucial in the field which is resource-intensive as deep learning, especially nowadays large language model exploration.
What are you hoping to do upon graduation?
I hope to be an assistant professor after graduation, and I am on the job market this year.
(For papers) What is the major improvement made in this work? What consequences does this improvement have for the field in general?
The major improvement made in this work is the development of a Structural Linearized Graph Convolutional Network (LinGCN) that optimizes the performance of Homomorphically Encrypted (HE) based GCN inference, reducing multiplication depth and addressing HE computation overhead.
This improvement has significant consequences for the field in general, as it enables the deployment of GCNs in the cloud while preserving data privacy. Additionally, the proposed framework can be applied to other machine learning models besides GCNs, making it a valuable contribution to the field of privacy-preserving machine learning.
Shaoyi Huang
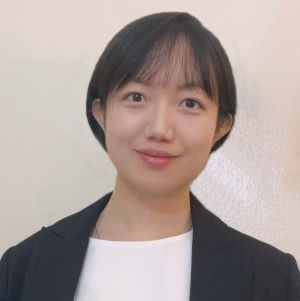
"Attending this year's conference would significantly enrich my experience, providing more opportunities to engage with experts in the field, strengthen my professional network, and enhance my prospects for a future faculty position. Therefore, receiving support from CACC for this trip would be invaluable to my career, helping me realize my dream''.